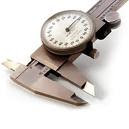
We use — and sometimes overuse — metrics to quantify profitability, return, productivity, efficiency, quality, etc.
But the metrics we use, should provide an objective score of results.
Should provide an objective score, but not always. Here’s an example.
I was in consulting at manufacturing at a production facility. Productivity was all-important, driving a number of performance and financial results. But productivity could be measured in many different ways.
Take throughput, a commonly used productivity metric. Throughput reflects the number of units produced per some period of time. If you produce 29,000 widgets in 8 hours, your throughput is 3,625 widgets/hour. Clean and simple.
Take throughput, a commonly used productivity metric. Throughput reflects the number of units produced per some period of time. If you produce 29,000 widgets in 8 hours, your throughput is 3,625 widgets/hour. Clean and simple.
Also misleading. In that business, throughput was almost meaningless as a metric.
Why? Say you manage a call center and the number of calls a sales rep makes is a key metric. (You assume the more calls made the more sales since in your business sales is in large part a numbers game.) Because your reps are cold calling prospects, call duration ranges from seconds to twenty minutes or more. Measuring call throughput over a long period of time — say, the average number of calls made per month — could help smooth out natural variation in call length and provide a relatively accurate reflection of a salesperson’s call productivity.
Why? Say you manage a call center and the number of calls a sales rep makes is a key metric. (You assume the more calls made the more sales since in your business sales is in large part a numbers game.) Because your reps are cold calling prospects, call duration ranges from seconds to twenty minutes or more. Measuring call throughput over a long period of time — say, the average number of calls made per month — could help smooth out natural variation in call length and provide a relatively accurate reflection of a salesperson’s call productivity.
Maybe.
As a short-term metric, though, throughput results could be very misleading. The same was true at the plant above. The product was the printing of books. They ran thousands of book titles a year of widely different run lengths. Some run quantities were under 2k, others over 500k. Running a number of jobs with small quantities during a shift automatically decreased throughput, since changing from job to job took time. The more jobs run in a day, the lower the throughput — even if the crew was incredibly efficient.
So we measured run and makeready results separately, using two simple formulas:
As a short-term metric, though, throughput results could be very misleading. The same was true at the plant above. The product was the printing of books. They ran thousands of book titles a year of widely different run lengths. Some run quantities were under 2k, others over 500k. Running a number of jobs with small quantities during a shift automatically decreased throughput, since changing from job to job took time. The more jobs run in a day, the lower the throughput — even if the crew was incredibly efficient.
So we measured run and makeready results separately, using two simple formulas:
Makeready average = total makeready time / number of makereadies
Run average = total books produced / total run hours
For example, during a shift a crew may have run 3,800 books per hour at an average makeready of 24 minutes.
Sound good? Not so fast. Comparing results, either between crews or over time, was a problem. Which is better:
3,800 books per hour and a 24 minute makeready average?
Or
Sound good? Not so fast. Comparing results, either between crews or over time, was a problem. Which is better:
3,800 books per hour and a 24 minute makeready average?
Or
3,750 books per hour and a 22 minute makeready average?
If you can’t answer the question, don’t worry, we couldn’t either: not without doing some maths, whether a slower run average and a faster makeready average was better than a faster run average and a slower makeready average depended on each crew’s total number of makereadies.
Worse, the crews knew that, so they would manipulate results, writing down slower or longer makeready times as they saw fit to manipulate results. While a shift always added up to 12 hours, how time was actually spent during that 12 hours was open to manipulation.
Instead of attempting (and probably failing) to enforce more accurate reporting, we created a metric we called adjusted books/hour (ABH).
Here’s how it worked:
Our makeready goal was 20 minutes, so every time a crew performed a makeready they got credit for a 20-minute makeready, regardless of how long that makeready actually took. If they had 3 makereadies during a 12-hour shift they got 60 minutes of makeready time. The remaining time was considered run time and was divided into the total books produced to determine ABH.
Here’s the formula:
ABH = total books / (total run time - (number of makereadies times 20 minutes))
Here’s how it worked:
Our makeready goal was 20 minutes, so every time a crew performed a makeready they got credit for a 20-minute makeready, regardless of how long that makeready actually took. If they had 3 makereadies during a 12-hour shift they got 60 minutes of makeready time. The remaining time was considered run time and was divided into the total books produced to determine ABH.
Here’s the formula:
ABH = total books / (total run time - (number of makereadies times 20 minutes))
Here’s an example. Say a crew had 6 makereadies and ran 29,000 books during an 12-hour shift. 6 makereadies times the makeready credit of 20 minutes equals 2 hours of makeready time. That reduces the total run time to 10 hours, and 10 into 29,000 equals 2,900 ABH.
The ABH metric gave us a straightforward way to evaluate performance trends and compare crew-to-crew performance. If crews performed makereadies faster than 20 minutes, great — they had more run time available and could run more books. If their makereadies took longer their ABH suffered since they had less actual time time to run books.
(Keep in mind we tracked actual performance on makereadies so we could spot areas for improvement; we just used ABH as an apples to apples metric.)
Take a look at what you measure:
Do some metrics leave room for interpretation?
Can some metrics be manipulated by how and when data is collected?
Do your metrics truly measure what is important to your business?
If not, think of ways to create your own metrics — especially if custom metrics make it easier for employees to evaluate their performance.
Measuring is important, but measuring what you need to measure — and measuring it the right way — is critical.
(Keep in mind we tracked actual performance on makereadies so we could spot areas for improvement; we just used ABH as an apples to apples metric.)
Take a look at what you measure:
Do some metrics leave room for interpretation?
Can some metrics be manipulated by how and when data is collected?
Do your metrics truly measure what is important to your business?
If not, think of ways to create your own metrics — especially if custom metrics make it easier for employees to evaluate their performance.
Measuring is important, but measuring what you need to measure — and measuring it the right way — is critical.
No comments:
Post a Comment